We sometimes forget the purpose of our work. In healthcare, the goal to make a positive impact on patients is present every day. This was the goal of the introduction of the first AI system in breast cancer screening… in 1998. Over the past 20 years, and especially with the breakthroughs in deep learning, the performance of such systems has improved and the time to design them has been greatly reduced. But what are the benefits to the patient? We illustrate two important ones.
The ultimate goal for any patient is the fastest possible recovery. With serious illnesses such as cancer, this is only possible when the diagnosis is made at an early stage of the disease. Do you believe that the primary benefit of AI is improved efficiency and speed of diagnosis by analyzing patient data to detect abnormalities? Make no mistake, an abnormality is not synonymous with pathology. There are therefore too many biopsies taken to find problem cases. It is therefore preferable to have an AI that, in addition to detecting an abnormality, can provide a prediction on its malignancy and thus justify a biopsy or other physical test. Nothing is perfect, but by combining a test using AI on the data and a physical test, we increase the reliability of the diagnosis.
Because each individual is different, the levels of response and side effects are varied. Once the disease is detected, the adage that the right treatment, for the right patient, at the right time, makes sense with the increased availability of personalized medicine.
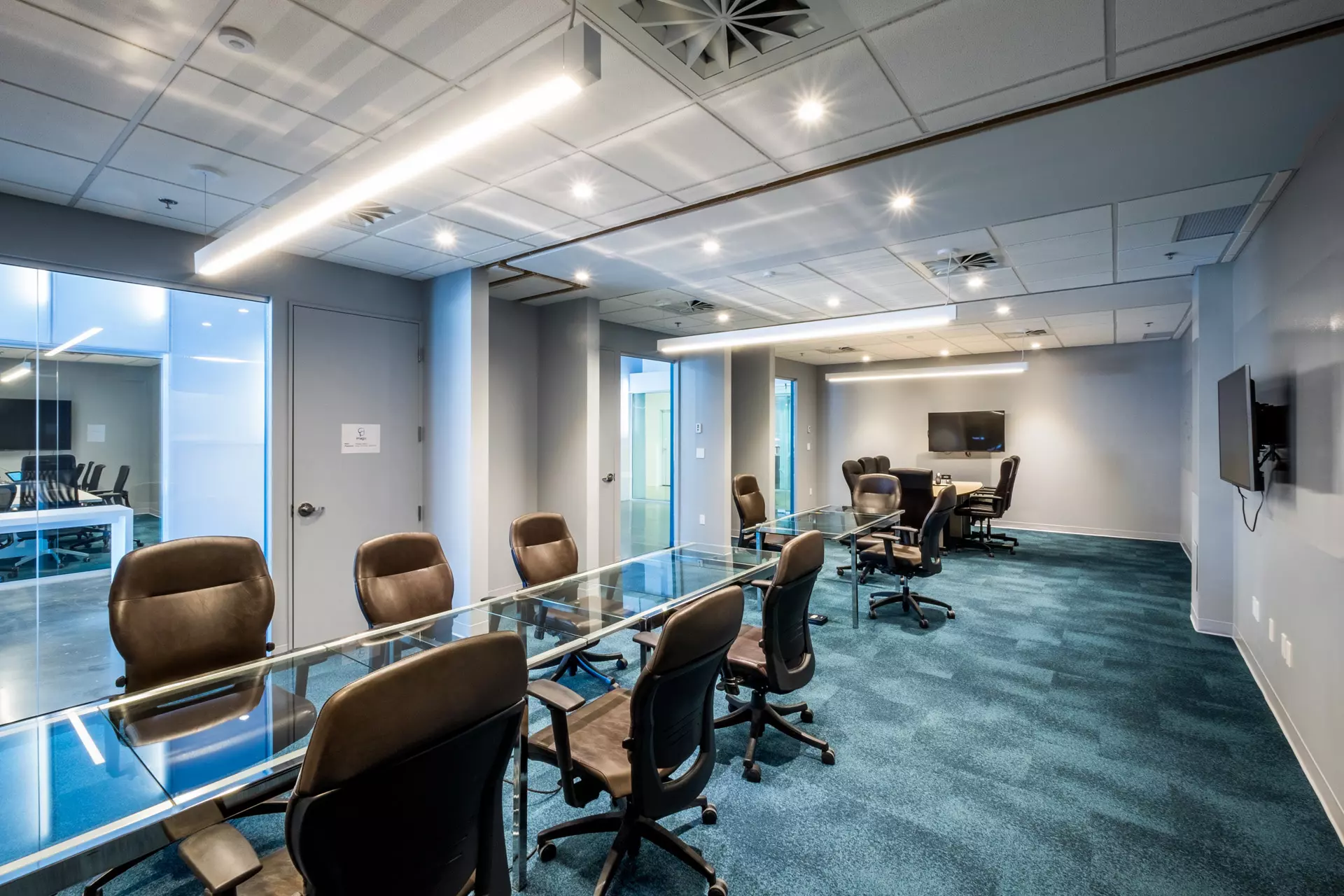
The second benefit of AI for patients is to better direct its therapeutic path to maximize a set that should be variable depending on the patient, through quality of life, survival, side effects, etc. AI is therefore essential for better patient care. Many solutions could have benefited patients who unfortunately did not make it to the hospital due to a lack of alignment of benefits to the 4 Ps: Patient, Payer, Provider and Practitioner.
Indeed, a lack of optimization of results for the payer will limit its marketing; the appropriate initiation of treatment by a Pharma (or medical device manufacturer) will promote its inclusion in the supply cycle and ultimately the solution must be useful to the already overburdened practitioner.
But designing these AI solutions requires patient data to train models to detect these pathologies or predict the response to a treatment. Is it possible to generate this common good without infringing on patients’ privacy? A simple solution is to keep the data at the hospitals, train the models locally on this data and combine the learning from several hospitals. Thus, data is neither shared nor studied by humans – only the result of learning or new hypotheses are shared. This is privacy by design through what is called federated learning.
This type of learning also makes it possible to take into account the genetic diversity, treatments and equipment that exist in several hospitals and that are representative of the population. Practitioners must also be autonomous both in the development of these AI tools and in their use to ensure adoption. These principles of privacy, diversity and autonomy are at the heart of the Montreal Declaration on Responsible AI. At Imagia, we apply them by design, but it is only through the collaboration of an ecosystem that new medical breakthroughs for patients will emerge.